Table of Contents
The Power of Data: Leveraging Big Data Analytics for Business Success
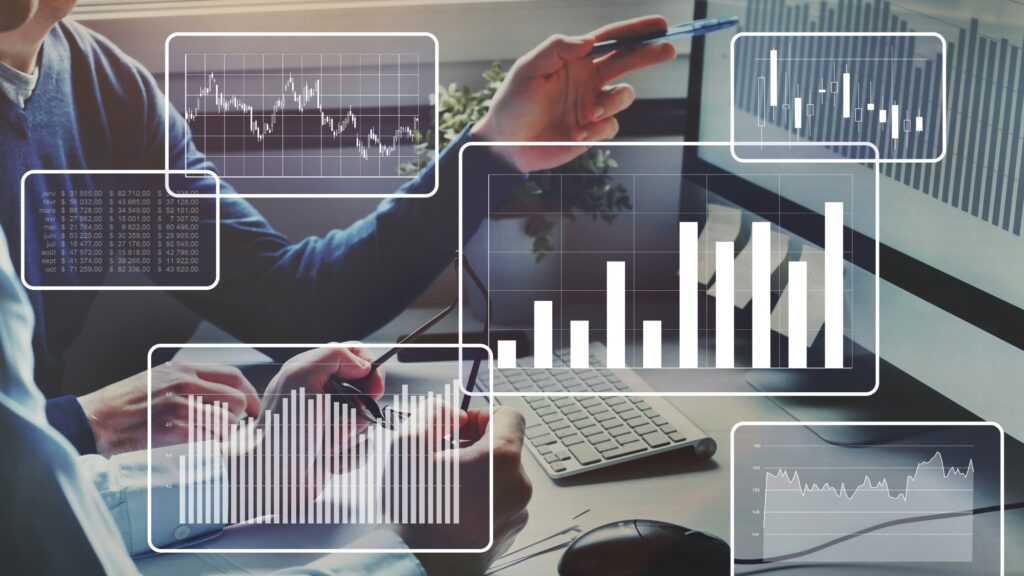
In today’s digital age, data is being generated at an unprecedented rate. From customer interactions to online transactions, every action we take leaves behind a trail of data. However, the real value lies in how businesses can harness this data and leverage it to gain insights, make informed decisions, and drive growth. This is where big data analytics comes into play.
Understanding Big Data Analytics
What is big data analytics?
Big data analytics refers to the process of examining large and complex data sets to uncover hidden patterns, correlations, and other valuable insights. It involves leveraging advanced technologies and algorithms to extract meaningful information from data that would otherwise be overwhelming or difficult to analyze manually.
The Benefits of Big Data Analytics for Businesses
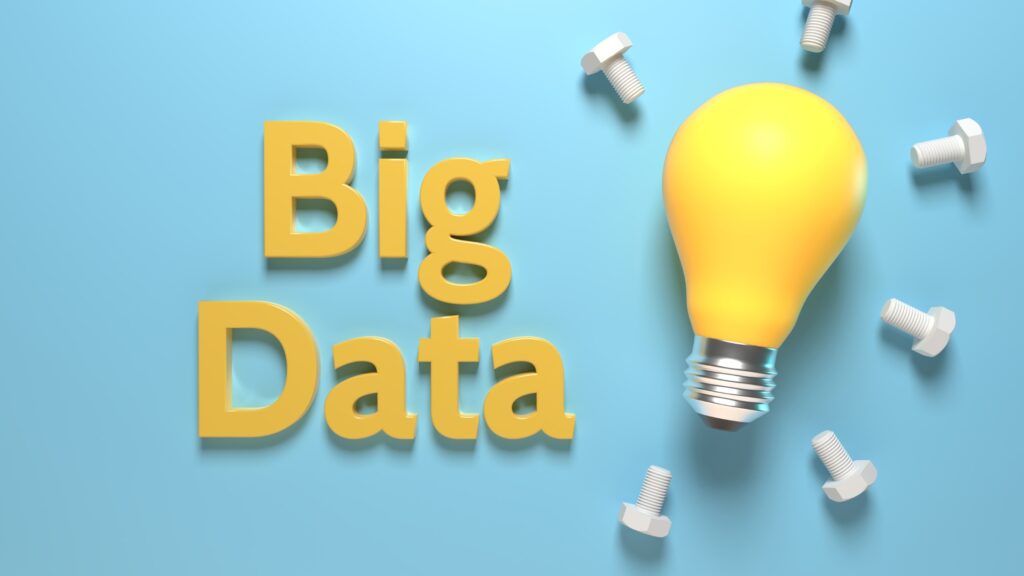
1. Enhanced Decision-Making
Utilizing big data analytics enables businesses to make more informed decisions. By analyzing large volumes of structured and unstructured data, businesses can gain valuable insights into customer behavior, market trends, and competitive landscapes. These insights serve as a foundation for strategic planning and informed decision-making.
2. Improved Operational Efficiency
In the ever-evolving world of business, staying competitive requires more than just providing excellent products or services. Operational efficiency is the linchpin that can often make the difference between thriving and merely surviving. Big data analytics offers businesses an invaluable tool to not only identify inefficiencies but to also streamline processes and minimize downtime, ensuring operations run like a well-oiled machine.
Identifying Inefficiencies:
Every organization, regardless of its size or industry, deals with operational inefficiencies. These bottlenecks, delays, or redundancies can hinder productivity and profitability. Big data analytics acts as a magnifying glass, enabling businesses to scrutinize every aspect of their operations. From production workflows to supply chain logistics and customer service interactions, data-driven insights reveal precisely where inefficiencies lie.
Streamlining Processes:
Once identified, these inefficiencies become targets for improvement. Big data analytics helps organizations redesign processes and workflows to eliminate bottlenecks and reduce friction points. For instance, in manufacturing, real-time data analysis can optimize production schedules, ensuring resources are allocated efficiently, and products are delivered on time. In the supply chain, data-driven insights enable companies to track inventory levels accurately, preventing overstocking or stockouts and ultimately reducing costs.
Minimizing Downtime:
Downtime is the enemy of productivity and profitability. Big data analytics empowers organizations to predict and prevent downtime events. By analyzing historical data and monitoring equipment performance in real time, businesses can schedule maintenance proactively, minimizing disruptions to production or service delivery. This not only saves time but also prevents costly breakdowns.
Anticipating Demand and Resource Allocation:
In the age of data, understanding market demand is no longer a guessing game. Businesses can leverage big data to anticipate shifts in demand, responding with agility. For example, retailers can adjust inventory levels based on predictive analytics, ensuring that they have the right products in stock when customers want them. This anticipatory approach to resource allocation not only enhances efficiency but also reduces waste.
Case Study: Supply Chain Optimization
Consider a global e-commerce giant that uses big data analytics to optimize its supply chain. By analyzing vast amounts of data, including customer orders, shipping times, and inventory levels, the company can make data-driven decisions about the most efficient shipping routes and fulfillment centers. This optimization results in faster delivery times, reduced shipping costs, and improved overall customer satisfaction.
In conclusion, the benefits of improved operational efficiency through big data analytics are multifaceted. It allows businesses to operate leaner, respond faster to market dynamics, and enhance overall customer satisfaction. The ability to identify inefficiencies, streamline processes, and minimize downtime empowers organizations to navigate today’s competitive landscape with agility and precision. As technology advances and data analytics techniques continue to evolve, the potential for operational optimization through big data analytics is limitless.
3. Personalized Customer Experiences
In an era where consumers have come to expect tailored interactions and offerings, the ability to provide personalized customer experiences has become a paramount competitive advantage. Big data analytics plays a pivotal role in deciphering the intricate nuances of customer behavior and preferences, allowing businesses to create experiences that resonate on an individual level.
The Power of Customer Segmentation:
At the heart of personalized customer experiences lies customer segmentation. Big data analytics allows businesses to categorize their customer base into distinct segments based on a myriad of factors such as demographics, purchase history, browsing behavior, and more. These segments act as a foundation for crafting customized marketing strategies, product recommendations, and communication approaches.
Targeted Marketing Campaigns:
Understanding your customer segments empowers businesses to create highly targeted marketing campaigns. Rather than employing a one-size-fits-all approach, companies can tailor their messages and promotions to specific customer groups. For example, a clothing retailer can send different email campaigns featuring winter coats to customers in colder regions and swimsuits to those in warmer climates. This level of personalization not only boosts the relevance of marketing efforts but also increases the likelihood of conversion.
Personalized Recommendations:
Big data analytics is the driving force behind the personalized product recommendations we encounter in e-commerce, streaming services, and even news websites. By analyzing a customer’s past purchases, browsing history, and preferences, businesses can suggest products or content that align with their interests. This not only enhances the customer’s shopping or viewing experience but also encourages cross-selling and upselling opportunities.
Tailored Offers and Loyalty Programs:
Businesses can leverage big data insights to design tailored offers and loyalty programs. These incentives are precisely customized to reward specific customer behaviors or preferences. For instance, a coffee shop can offer a free pastry to a customer who consistently orders a certain type of coffee. These personalized gestures not only strengthen customer loyalty but also drive repeat business.
Improving Customer Satisfaction:
Ultimately, personalized customer experiences lead to higher levels of customer satisfaction. When customers feel that a business understands their needs and values their preferences, they are more likely to remain loyal and become brand advocates. Positive interactions founded on personalization foster a sense of connection and trust between the customer and the brand.
Case Study: E-Commerce Personalization
Consider an e-commerce giant that employs big data analytics to personalize the shopping experience. By analyzing a customer’s browsing history, purchase behavior, and product preferences, the platform generates a “Recommended for You” section. This curated selection showcases products tailored to the individual customer’s taste. As a result, customers are more likely to discover and purchase items that resonate with their interests, leading to increased sales and customer satisfaction.
In conclusion, the ability to provide personalized customer experiences through big data analytics is no longer a luxury but a necessity in the modern business landscape. It not only boosts customer satisfaction and loyalty but also drives revenue growth by improving conversion rates and cross-selling opportunities. As technology continues to advance, the potential for even more sophisticated personalization will only expand, further cementing big data analytics as a cornerstone of customer-centric strategies.
4. Risk Management and Fraud Detection
In the intricate landscape of modern business, managing risks and safeguarding assets are paramount concerns. Big data analytics stands as a formidable ally in these endeavors, offering an arsenal of tools and techniques to identify, assess, and mitigate risks in real-time, while also providing robust fraud detection mechanisms.
Real-time Risk Identification:
The business environment is dynamic, and risks can materialize swiftly. Big data analytics equips organizations with the ability to monitor vast data streams in real-time, serving as an early warning system. By continuously scrutinizing data from various sources, such as financial transactions, market fluctuations, and operational metrics, businesses can swiftly detect anomalies and deviations from expected patterns. This proactive approach allows for timely intervention and risk mitigation.
Fraud Detection Precision:
In an era where cyber threats and fraudulent activities have reached unprecedented sophistication, businesses require equally advanced tools for protection. Big data analytics-driven fraud detection systems are designed to recognize and respond to suspicious behavior. These systems scrutinize transactions, user behaviors, and access patterns, flagging any irregularities that may indicate fraudulent activities. The granularity of analysis is such that even subtle deviations from established norms trigger alerts for further investigation.
Minimizing Financial Losses:
The financial consequences of fraud can be devastating, ranging from direct monetary losses to damage to reputation and customer trust. Big data analytics acts as a financial guardian, minimizing losses by swiftly detecting and responding to fraudulent activities. By preventing or mitigating fraud, businesses can protect their bottom line and preserve the trust of their customers.
Adaptive Risk Assessment:
The power of big data analytics extends to predictive modeling and machine learning algorithms. These tools analyze historical data and continuously learn from new information to improve risk assessment. By identifying patterns and trends, businesses can anticipate potential risks before they escalate. For instance, a bank may employ machine learning to detect unusual spending patterns on a credit card, alerting the customer and blocking potentially fraudulent transactions.
Compliance and Regulatory Adherence:
In an increasingly regulated business environment, compliance with industry standards and regulations is non-negotiable. Big data analytics facilitates compliance efforts by monitoring data for adherence to regulatory requirements. It helps organizations ensure that they are operating within legal boundaries, thereby avoiding costly fines and penalties.
Case Study: Financial Services Fraud Prevention
Consider a financial services institution leveraging big data analytics to prevent fraudulent activities. The institution employs machine learning algorithms to analyze transaction data, customer profiles, and historical fraud patterns. When a transaction exhibits characteristics indicative of potential fraud, the system automatically triggers alerts, allowing the institution to investigate and take corrective action promptly. This proactive approach saves the institution and its customers from significant financial losses.
In conclusion, big data analytics has become the linchpin of modern risk management and fraud detection efforts. Its ability to analyze vast volumes of data in real-time, coupled with predictive capabilities, not only minimizes financial losses but also safeguards reputation and customer trust. As risks continue to evolve in complexity, businesses that embrace the power of big data analytics are better equipped to navigate these challenges and protect their interests in a rapidly changing business landscape.
5. Innovation and Product Development
In the relentless pursuit of growth and competitiveness, innovation becomes the lifeblood of businesses. Big data analytics, with its ability to decipher market dynamics and customer behavior, serves as a wellspring of inspiration and knowledge, propelling innovation and guiding the development of cutting-edge products and services.
Market Insight for Strategic Innovation:
Understanding market trends is a cornerstone of successful innovation. Big data analytics empowers businesses to monitor and analyze market dynamics in real-time, providing invaluable insights into consumer preferences, emerging trends, and competitive landscapes. Armed with this information, organizations can identify unmet needs and untapped opportunities, serving as the catalyst for innovative product or service development.
Customer-Centric Product Development:
In the era of data, customers’ voices resonate louder than ever. Big data analytics transforms customer feedback into actionable insights. By mining customer reviews, surveys, and social media interactions, businesses can gain a profound understanding of what customers truly desire and where they encounter pain points. This data-driven approach informs product development strategies, ensuring that new offerings align precisely with customer needs.
Competitor Intelligence:
Innovation is not solely about creating something entirely new; it’s also about staying ahead of the competition. Big data analytics allows businesses to monitor and analyze competitor activities, product launches, and market positioning. This competitive intelligence helps organizations identify gaps or weaknesses in the offerings of their rivals and seize opportunities to innovate and differentiate.
Optimizing Existing Products and Services:
Innovation isn’t limited to the creation of entirely new products or services. Big data analytics aids in the optimization of existing offerings. By analyzing customer usage patterns and feedback, businesses can identify areas for improvement, whether it’s enhancing features, refining user interfaces, or adjusting pricing strategies. These incremental innovations can strengthen customer loyalty and drive revenue growth.
Predictive Innovation:
Predictive analytics, a subset of big data analytics, allows businesses to anticipate future trends and customer demands. By analyzing historical data, organizations can develop models that forecast market shifts and customer preferences. This foresight enables businesses to innovate proactively, staying ahead of the curve rather than reacting to market changes.
Case Study: Streaming Media Platform
Consider a streaming media platform that utilizes big data analytics for innovation. By analyzing user data, including viewing habits, search queries, and content preferences, the platform identifies gaps in its content library. It then uses this information to acquire or produce new content tailored to user interests. This data-driven approach not only attracts new subscribers but also retains existing ones, as users find a wider range of content that resonates with their preferences.
In conclusion, the fusion of big data analytics and innovation has become an indomitable force in the business world. It enables organizations to spot opportunities, understand customer needs, and outmaneuver competitors. By leveraging data-driven insights, businesses can foster a culture of innovation that permeates every aspect of their operations, from product development to marketing strategies, and ensure continued growth and relevance in an ever-evolving marketplace.
Implementing Big Data Analytics
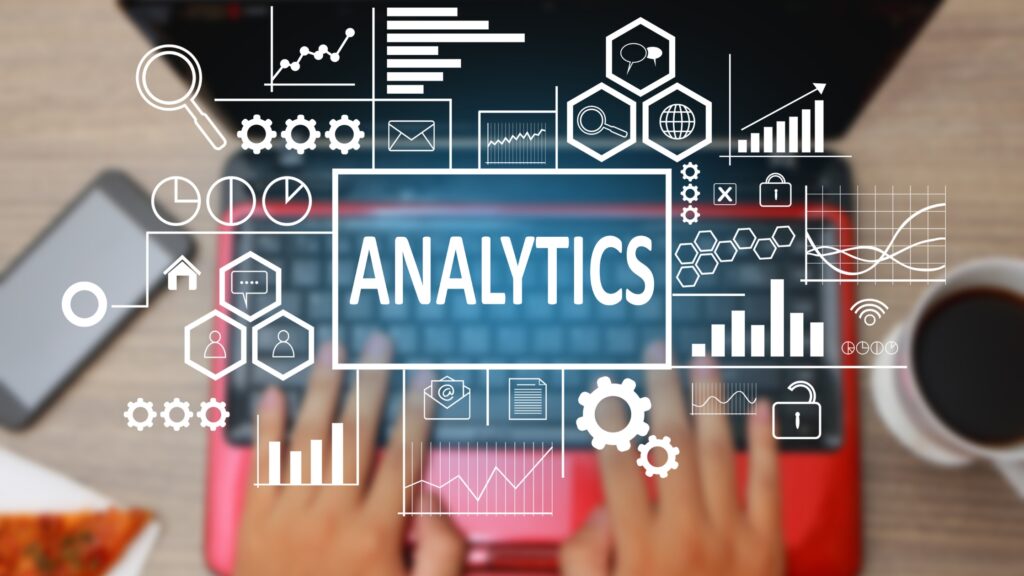
While the benefits of big data analytics are undeniable, implementing an effective analytics strategy requires careful planning and consideration. Here are a few steps to get started:
1. Define Business Objectives
Identifying specific goals and objectives is the first crucial step in any big data analytics initiative. Consider what you want to achieve through data analysis—whether it’s increasing revenue, reducing operational costs, enhancing customer satisfaction, or improving operational efficiency. These objectives will serve as your guiding stars throughout the analytics journey, ensuring that every action aligns with your overarching business goals.
The objectives should be specific and well-defined. Instead of a vague goal like “improve business,” it’s better to articulate a precise objective, such as “increase quarterly revenue by 15%,” “reduce customer churn by 20%,” or “optimize supply chain operations to reduce costs by 10%.” Specificity ensures that everyone involved understands the exact aim of the analytics effort.
2. Data Collection and Integration
This stage in implementing big data analytics is all about acquiring and consolidating the data you need for your analysis. It’s a crucial step because the quality and relevance of the data you collect will significantly impact the effectiveness of your analytics strategy.
Determining the Right Data Sources:
First and foremost, you need to decide where your data will come from. Data can be categorized into two main types: internal and external sources.
Internal Data Sources: These are the data generated and collected within your organization. They typically include:
- Sales Records: Information about products or services sold, transaction details, and customer information.
- Customer Data: Details about your customers, including demographics, preferences, purchase history, and interaction history.
- Operational Metrics: Data related to your day-to-day business operations, such as production output, inventory levels, and employee performance.
External Data Sources: These are data that originates from outside your organization but can be highly valuable for your analytics efforts. External data sources can include:
- Social Media Data: Information from platforms like Facebook, Twitter, or LinkedIn, which can provide insights into customer sentiment, trends, and engagement.
- Industry Reports: Reports and publications relevant to your industry, which may contain market trends, competitor information, and consumer behavior data.
- Market Research: Data gathered through surveys, studies, or research conducted by external agencies or firms, providing insights into consumer preferences and market dynamics.
- Sensor Data: In some cases, businesses collect data from sensors or IoT (Internet of Things) devices, which can include environmental data, machine performance metrics, or even data from connected products.
Relevance to Your Objectives:
The key to successful data collection is relevance to your specific objectives. It’s not about collecting as much data as possible; it’s about gathering the right data that aligns with your goals. For instance, if your objective is to improve customer satisfaction, data related to customer feedback and support interactions would be highly relevant. On the other hand, if you’re aiming to optimize manufacturing processes, data on machine performance and production output would be more critical.
Comprehensive View of Your Business Environment:
By collecting data from both internal and external sources, you gain a comprehensive view of your business environment. Internal data provides insights into your organization’s performance and customer interactions, while external data offers broader market context and competitive intelligence. Combining these data sources allows you to make more informed decisions, spot trends, and respond effectively to changes in your industry.
In essence, data collection and integration are about building the foundation for your analytics endeavors. It’s the process of gathering the building blocks, ensuring that they fit together seamlessly, and setting the stage for the analysis and insights that will follow.
3. Data Cleansing and Preprocessing
Imagine raw data as a block of uncut gemstone—it has potential, but it’s not yet ready to shine. Data cleansing and preprocessing are the processes of refining this raw data to make it accurate, reliable, and consistent—like cutting and polishing that gemstone to reveal its true value.
Why Data Isn’t Perfect:
Raw data, when collected, often contains imperfections. These imperfections can be errors, duplicate entries, or information that’s not relevant to your analysis. For example, in a customer database, you might find misspelled names, duplicate customer records, or incomplete contact information.
The Data Refinement Process:
Data Validation: This step checks data for accuracy and consistency. It identifies and corrects errors, ensuring that data is in the right format and adheres to defined standards. For instance, validating email addresses to make sure they follow the correct format.
Data Cleaning: Data cleaning involves removing duplicates and correcting errors or inconsistencies. It’s like removing duplicate gemstone pieces or polishing out imperfections. For example, merging duplicate customer records.
Data Transformation: Sometimes, data needs to be transformed into a different format for analysis. This can involve converting units of measurement or aggregating data. For instance, converting sales figures from different currencies to a common currency for analysis.
Data Normalization: Data may come from various sources and have different scales. Normalization standardizes data to ensure fair comparisons. For example, scaling customer satisfaction scores from 1-10 to a standardized range of 0-1.
The Importance of High-Quality Data:
Data quality is essential because the insights and decisions drawn from data are only as good as the data itself. Clean and well-preprocessed data ensures that your analysis is based on a solid foundation, preventing errors or skewed results. It’s like ensuring your gemstone is flawlessly cut and polished to reveal its true brilliance.
In summary, data cleansing and preprocessing are essential steps to refine raw data, making it accurate, reliable, and consistent. This refined data is the bedrock for deriving meaningful insights and making informed decisions in your analytics endeavors. Just as a polished gem shines brighter, high-quality data illuminates the path to successful data-driven insights.
4. Data Storage and Infrastructure
Think of data storage and infrastructure as the solid foundation upon which your data analytics house is built. It’s the part of the process that ensures your data is not only accessible but also safe and ready for analysis, especially when dealing with large amounts of data.
Why It Matters:
When dealing with big data, you’re dealing with a vast amount of information. This data needs a proper home where it can be organized, stored securely, and accessed efficiently.
Building the Foundation:
Data Warehouse: This is like a centralized data repository designed for analytical processing. It’s structured to store and manage data efficiently, making it easier to retrieve and analyze. Think of it as a well-organized library for your data.
Cloud-Based Solutions: Cloud platforms offer scalable and flexible data storage options. It’s like renting space in a warehouse; you can expand or contract your storage space as needed, making it a cost-effective choice.
Data Management Tools and Technologies: These are like the tools in a workshop. They help organize, process, and maintain your data efficiently. Examples include database management systems, data lakes, and data governance tools.
Goals of Data Storage and Infrastructure:
The primary goal is to create an environment where data is:
Secure: Protecting your data from unauthorized access or loss is paramount. It’s like having strong locks and security systems in your storage facility.
Scalable: As your data grows, your storage should grow with it. Think of it as adding more floors to a building when you need more space.
Efficient: Retrieving and analyzing data should be quick and smooth. It’s like organizing your warehouse so that you can easily find and access what you need.
Why a Strong Foundation Matters:
Imagine a library with books scattered everywhere, no security, and slow access. It would be chaotic and inefficient. Similarly, without proper data storage and infrastructure, your analytics efforts can be chaotic, insecure, and slow. With the right foundation, you have a well-organized, secure, and efficient data environment ready for analysis.
In essence, data storage and infrastructure are like the solid ground upon which your data analytics activities stand. It’s the backstage that ensures everything runs smoothly, making your data readily available for analysis and decision-making while keeping it safe and sound.
5. Data Analysis and Visualization
Once you’ve gathered, cleaned, and stored your data, it’s time to turn it into valuable insights and share those insights effectively. This stage involves using various tools and techniques to analyze the data and then presenting those findings through data visualization.
Data Analysis:
Advanced Analytics Tools and Techniques: Think of these as your investigator’s toolkit. They include software, algorithms, and statistical methods that help you make sense of the data. For example, you might use predictive modeling to forecast future trends or machine learning to uncover complex patterns in customer behavior.
Types of Analysis: Data analysis can range from simple reporting, where you present basic facts and figures, to more advanced techniques like predictive analytics, which uses historical data to make future predictions. It’s like going from summarizing a book’s plot to predicting how the story will evolve.
Data Visualization:
Effective Communication: Data visualization is the art of turning numbers and statistics into visual stories. It’s like creating a picture book that makes complex information easy to understand. Graphs, charts, and infographics are common tools for data visualization.
Clarity and Understanding: Just as a picture is worth a thousand words, a well-designed data visualization can convey insights much more clearly than pages of raw data. It helps stakeholders, including non-technical ones, grasp the significance of the findings.
Why Data Analysis and Visualization Matter:
Insight Discovery: Data analysis reveals patterns, trends, and correlations in your data that might not be apparent at first glance. For example, it might uncover that certain products sell better during specific seasons.
Informed Decision-Making: These insights empower organizations to make informed decisions. For instance, based on data analysis, a retailer might decide to adjust their inventory to match seasonal demand, leading to cost savings.
Communication: Data visualization ensures that these insights are effectively communicated to stakeholders, whether it’s presenting findings to a board of directors or explaining trends to customers. It’s like turning raw data into a compelling story.
Example:
Imagine an e-commerce platform analyzing its customer data. They use advanced analytics to identify that customers who view product videos are more likely to make a purchase. They create a data visualization showing this trend with a graph. This clear visualization communicates the insight effectively to marketing teams, helping them prioritize creating more product videos.
In summary, data analysis and visualization are the steps where data transforms into actionable insights and clear communication. They’re the process of digging into the data to discover hidden treasures and then crafting a visual narrative to share those treasures with the world, ultimately driving informed decision-making and understanding.
6. Continuous Monitoring and Optimization
Big data analytics isn’t a one-time endeavor; it’s a continuous journey. Just as a ship needs regular navigation adjustments to reach its destination, your analytics efforts require ongoing attention and refinement.
The Ongoing Process:
Continuous Monitoring: Think of this as regularly checking the health of your analytics systems. You keep an eye on data sources, algorithms, and models to ensure everything is running smoothly. It’s like regularly inspecting the engine of a car to ensure it’s performing optimally.
Evaluation: This involves assessing the effectiveness of your analytics initiatives. Are you achieving your objectives? Are the insights still relevant? Evaluation is akin to reviewing your progress and adjusting your route if needed during a long road trip.
Refinement: Just as software gets updates, your analytics models and strategies need improvements. Refinement might involve adjusting algorithms, expanding data sources, or fine-tuning your analytics methods. It’s like enhancing a recipe based on feedback to make it even tastier.
Adaptation to New Insights and Changing Needs:
Business landscapes evolve, customer preferences shift, and new data sources emerge. Continuous monitoring and optimization ensure that your analytics efforts remain aligned with these changes. It’s like recalibrating your compass when the terrain changes during a hiking trip.
Why It Matters:
Staying Relevant: The insights and strategies that worked yesterday might not be as effective tomorrow. Continuous monitoring and optimization keep you up-to-date and relevant in a rapidly changing environment.
Efficiency: Regular checks and improvements make your analytics efforts more efficient. You avoid wasted resources on outdated approaches and focus on what truly matters.
Maximizing Benefits: Businesses invest time and resources in analytics to gain a competitive edge and make better decisions. Continuous optimization ensures you’re maximizing the benefits of that investment.
Example:
Consider an e-commerce platform that uses analytics to optimize its advertising campaigns. After implementing analytics, they notice an increase in click-through rates. However, after a few months, they see a decline in engagement. Continuous monitoring reveals that customer preferences have shifted. They adapt their campaigns to align with these new preferences, leading to renewed success.
In conclusion, continuous monitoring and optimization are the compass and fuel for your analytics journey. They ensure that your analytics efforts remain effective, efficient, and aligned with your evolving business needs, ultimately driving ongoing success and competitiveness in a dynamic data-driven world.
Conclusion
The power of data lies not in the sheer amount of information we have but in how we harness and analyze it. Big data analytics provides businesses with the ability to gain valuable insights, make informed decisions, and drive growth. By leveraging the power of data, businesses can optimize operations, enhance customer experiences, mitigate risks, and uncover new opportunities. As the digital landscape continues to evolve, businesses that embrace big data analytics are poised to thrive in an increasingly data-driven world.